Emilie Wedenborg
_______________________________________________________
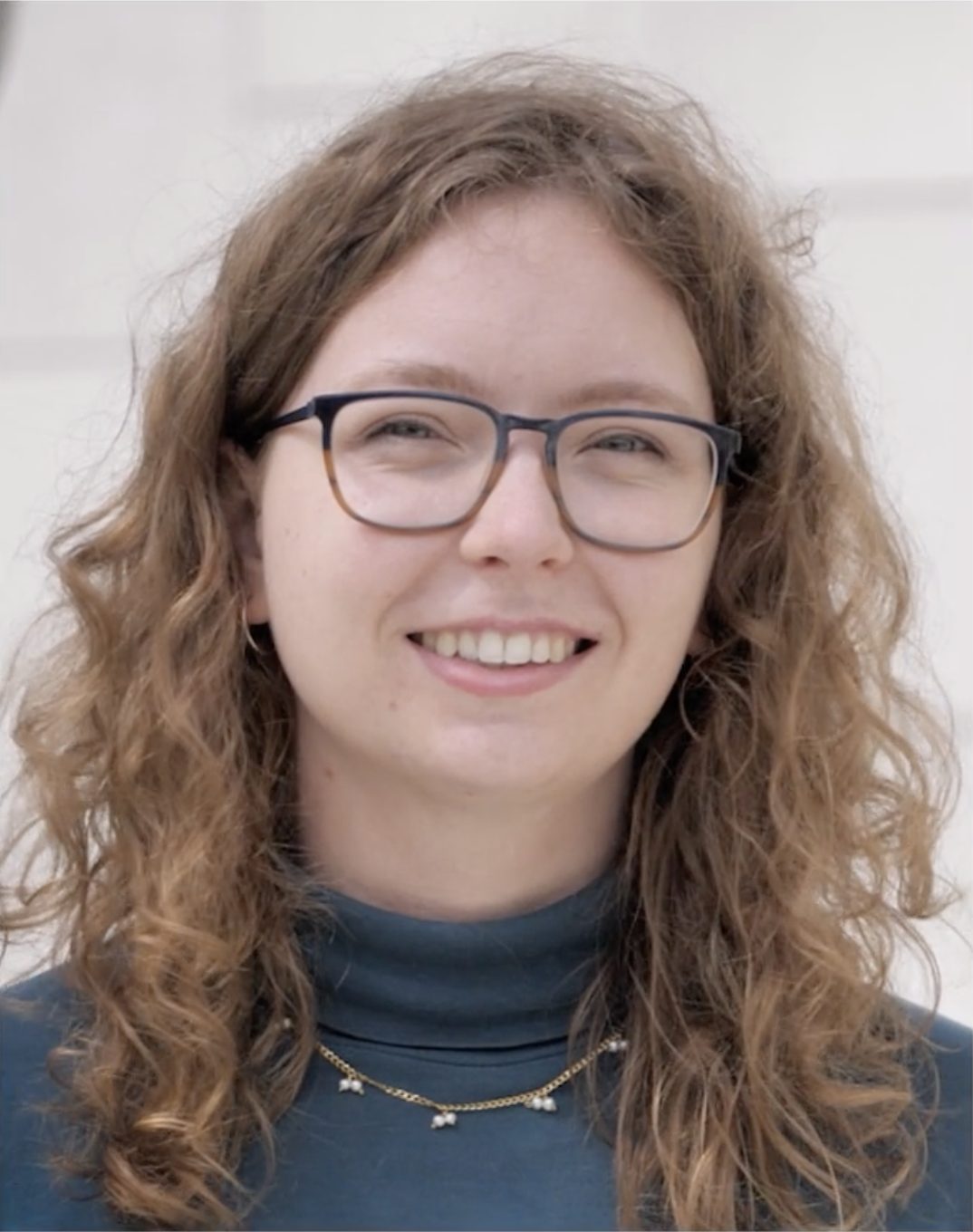
MSc in Mathematical Modelling and Computing
PhD @ Technical University of Denmark
Abstract
Discovering Polytopes in High Dimensional, Heterogeneous Datasets using Bayesian Archetypal Analysis
Real World Data (RWD), such as electronic medical records, national health registries, and insurance claims data provide vast amounts of high granularity heterogeneous data. An international standard (OMOP) has been developed for health data and accelerating evidence generation from RWD. EU has recently adopted the same standard for the European Health Data & Evidence Network (EHDEN), the largest federated health data network covering more than 500 million patient records. This allows standardization of datasets across institutions in 26 different countries, but a major data science challenge remains on how to tackle the volume and complexity of multimodal data of such magnitude.
The aim is to develop easily human interpretable tools to analyse RWD to extract distinct characteristics enabling new discoveries. The project includes a key industrial collaborator, H. Lundbeck A/S, that will provide additional guidance, contacts, and access to large sets of RWD in the OMOP format.
The project will focus on a prominent data science methodology called Archetypal Analysis characterized by identifying distinct characteristics, archetypes, and how observations are described in terms of these archetypes, thereby defining polytopes in high-dimensional data. This project will develop tools for uncovering such polytopes in large, high-dimensional, heterogenous, noisy, and incomplete data. We will develop Bayesian modeling approaches for uncertainty and complexity characterization, data fusion for enhanced inference, and deep learning methods to uncover disentangled polytopes.
The tool will advance our understanding of RWD and will accelerate real world evidence generation through the identification of patterns in terms of archetypes. Furthermore, trade-offs within archetypes can fuel personalized medicine by defining a profile of the individual patient in terms of a soft assigned spectrum between archetypes. We hypothesize this characterization has important use advancing our understanding of subtypes and comorbidities within different neurological and psychiatric disorders.