Richard Michael
_______________________________________________________
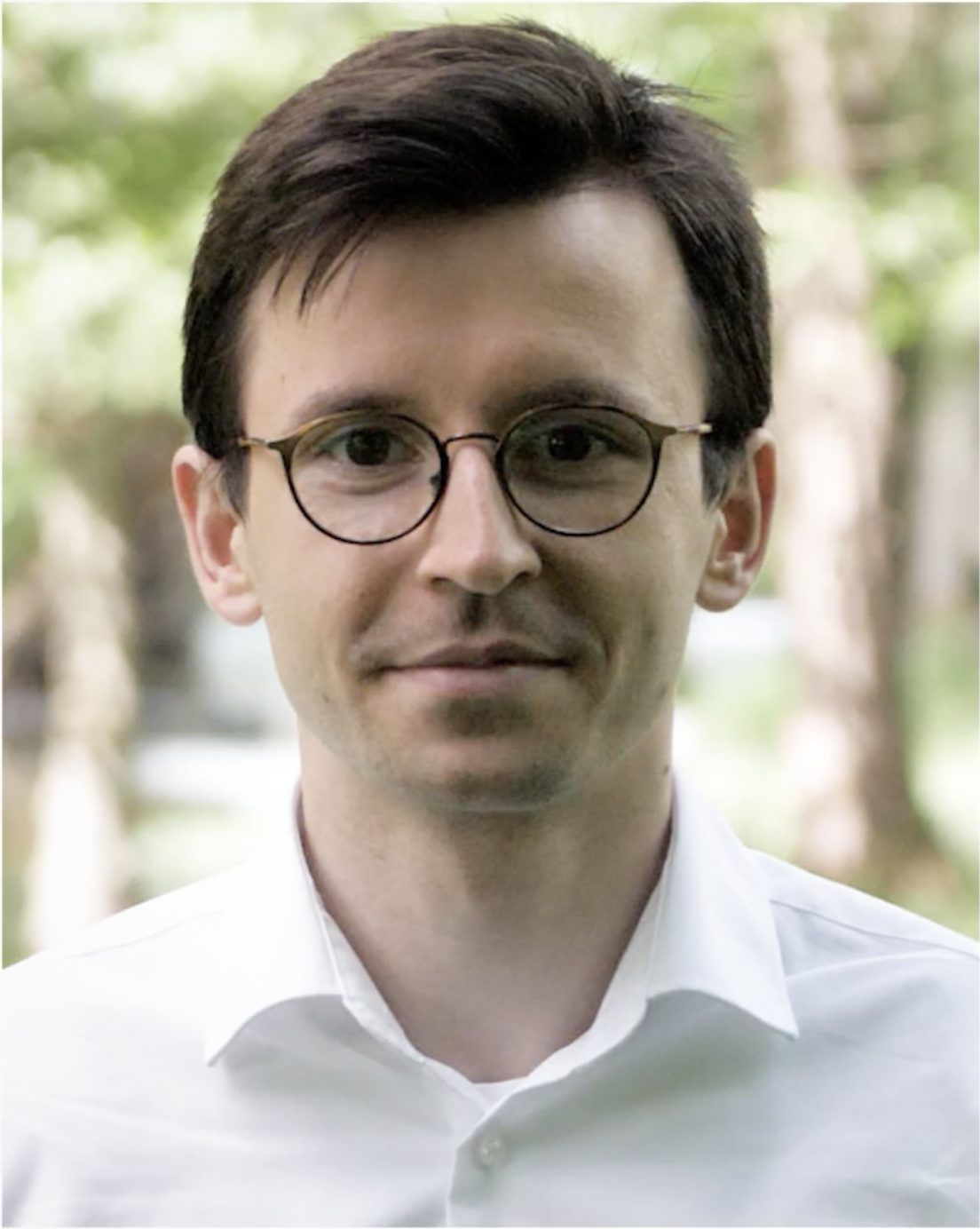
MSc in Bioinformatics
PhD @ University of Copenhagen
Abstract
Principled Bayesian Optimization and scientific ML for Protein Engineering
Protein Engineering has a wide range of applications from biotechnology to drug discovery. The design of proteins with the intended properties entails a vast discrete search-space and while we have computational representations and experimental observations available, we lack the methods to adequately combine all available information. In this proposed work we use the state of the art probabilistic optimization and propose a novel machine learning method: principled Bayesian Optimization on latent representations applied to protein variants.
We utilize abundant cheap experimental observations together with various latent information from deep stochastic models. We optimize the target function on a large data-set of lower quality experiments with respect to very scarce high quality experimental candidates through dual output Gaussian Processes. This method promises to predict the highest scoring variants given abundant noisy assay data. The goal is to significantly improve predictions of protein variant candidates with respect to intended function. This project is a collaboration between the Bio-ML group at the Department of Computer Science and the department of Chemistry under joint supervision at the University of Copenhagen.
The successful outcome of the research project would allow us to reduce required experimental time and resources through better computational protein variant proposals. We propose to achieve this by incorporating different data sources and account for epistemic and aleatoric noise.